Statistics Indian Statistical Service Part 3 Question-7 Solved Solution
- SOURAV DAS
- Mar 24, 2023
- 8 min read
Updated: Apr 4, 2023
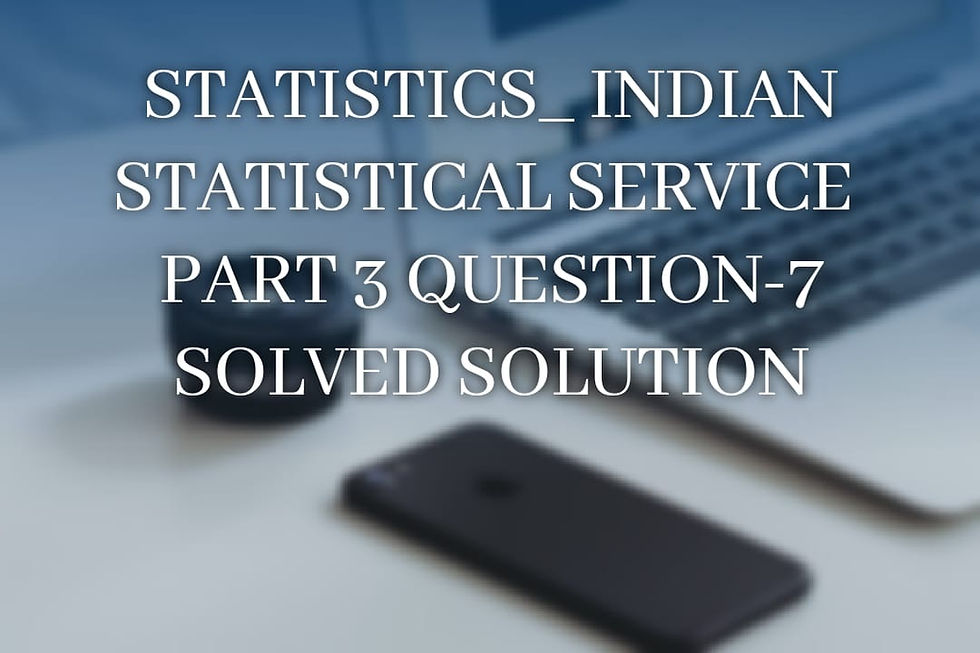
(i) Define endogenous variables, exogenous variables, and predetermined
variables.
Endogenous variables, exogenous variables, and predetermined variables are terms used in the context of econometrics, which is a branch of economics that deals with the statistical analysis of economic data.
Endogenous variables are those variables that are affected by other variables in the system being studied. They are typically the variables of interest in the analysis, as they are the ones that we want to understand and explain. For example, in a model of the labor market, the wage rate could be an endogenous variable because it is affected by other variables such as labor supply and demand.
Exogenous variables, on the other hand, are variables that are not affected by other variables in the system. They are typically used as explanatory variables in the analysis, as they can help us to understand the behavior of the endogenous variables. For example, in a model of the labor market, the unemployment rate could be an exogenous variable because it is not affected by the wage rate.
Predetermined variables are variables that are fixed at the time when the endogenous variables are determined. They are typically used to account for the fact that the endogenous variables are not independent of past values of themselves or other variables. For example, in a model of investment, the level of capital stock could be a predetermined variable because it is fixed at the time when investment decisions are made.
(ii) Consider the general structural form of simultaneous equations model and explain the problem of identification using its likelihood function.
The general structural form of a simultaneous equations model can be written as:
y = Ax + Bu + e
where y is a vector of endogenous variables, x is a vector of exogenous variables, u is a vector of other exogenous variables or random errors, A and B are matrices of coefficients, and e is a vector of random errors. This equation represents a system of equations where the endogenous variables are simultaneously determined.
The problem of identification in a simultaneous equations model arises because the coefficients of the model cannot be estimated without some additional assumptions or restrictions. This is because each endogenous variable in the system is determined by both the exogenous variables and the other endogenous variables, making it impossible to separately identify the effects of each variable on the others.
One approach to address the problem of identification is to impose exogeneity assumptions on some of the variables in the model. This means assuming that some of the variables are not affected by the other endogenous variables in the system. This assumption allows us to use those variables as instruments to estimate the coefficients of the other variables. However, this approach requires a priori knowledge of the variables that are exogenous, which may not always be available.
Another approach is to use a likelihood function to estimate the parameters of the model. The likelihood function is a function that measures the probability of observing the data given a set of parameters. However, in a simultaneous equations model, the likelihood function may have multiple local maxima, making it difficult to identify the true parameters of the model. This is because the likelihood function depends on the joint distribution of the endogenous variables, which is affected by the correlations among them. As a result, the likelihood function may not be globally concave, which means that there may be multiple sets of parameters that provide equally good fits to the data.
Overall, the problem of identification in a simultaneous equations model is a fundamental challenge in econometrics, and it requires careful consideration of the assumptions and restrictions imposed on the model to obtain reliable estimates of the parameters.
(iii) Determine the rank and order conditions for the identification of a particular equation.
In a simultaneous equations model, the identification of a particular equation requires that the equation be uniquely determined by the other equations in the system. This requires that the equation satisfies both rank and order conditions.
The rank condition requires that the coefficient matrix of the equation has full column rank. In other words, the number of linearly independent columns in the matrix should be equal to the number of endogenous variables in the equation. Mathematically, if we have a system of n equations and k endogenous variables in the equation of interest, then the rank condition is satisfied if and only if the rank of the coefficient matrix of the equation is k. If the rank condition is not satisfied, then the equation cannot be uniquely determined by the other equations in the system, and it is not identified.
The order condition requires that the equation has at least one exogenous variable that is not included in any of the other equations in the system. This variable is called an exclusion restriction, and it is necessary to identify the equation. If the equation does not have an exclusion restriction, then it is not identified.
In summary, for a particular equation in a simultaneous equations model to be identified, it must satisfy both the rank and order conditions. The rank condition ensures that the equation can be uniquely determined by the other equations in the system, while the order condition ensures that the equation has an exclusion restriction that allows it to be identified.
7.(b) Consider the following system of equations:
(i) = +
(ii) = + +
where y's are endogeneous and x's are predetermined variables. Check the identifiability of both the equations. If any of these two equations is identifiable, explain the method of two-stage least squares for estimating the parameters of that equation.
To check the identifiability of the two equations, we need to verify whether they satisfy the rank and order conditions.
For equation (1), we have:
y_1t = β_10 +β_11 y_2t + γ_11 x_1t + γ_12 x_2t + u_1t
This equation has two endogenous variables, y_1t and y_2t, and two predetermined variables, x_1t and x_2t. Therefore, the rank condition requires that the coefficient matrix of the equation has full column rank with two linearly independent columns. We can write the coefficient matrix as:
|β_11 γ_11 γ_12|
This matrix has full column rank if and only if β_11 is not equal to 0. If β_11 = 0, then the equation is not identified.
The order condition requires that the equation has at least one exogenous variable that is not included in any of the other equations in the system. In this case, both x_1t and x_2t appear in equation (1) and (2), so neither of them can serve as an exclusion restriction. Therefore, equation (1) is not identified.
For equation (2), we have:
y_2t = β_20 +β_21 y_1t + u_2t
This equation has one endogenous variable, y_2t, and one predetermined variable, y_1t. Therefore, the rank condition requires that the coefficient matrix of the equation has full column rank with one linearly independent column. We can write the coefficient matrix as:
|β_21|
This matrix has full column rank if and only if β_21 is not equal to 0. If β_21 = 0, then the equation is not identified.
The order condition requires that the equation has at least one exogenous variable that is not included in any of the other equations in the system. In this case, there is no predetermined variable in equation (2) that is not included in equation (1), so we cannot use an exclusion restriction to identify the equation.
Therefore, neither of the equations in the system is identified.
If equation (1) were identified, we could use the method of two-stage least squares (2SLS) to estimate its parameters. The 2SLS method involves two stages:
1. In the first stage, we regress the endogenous variable, y_2t, on the predetermined variables, x_1t and x_2t, and any other exogenous variables that are available. This gives us the predicted values of y_2t, denoted by ŷ_2t.
2. In the second stage, we regress y_1t on ŷ_2t, x_1t, x_2t, and any other exogenous variables that are available. This gives us the estimated coefficients of equation (1), including β_10, β_11, γ_11, and γ_12.
The 2SLS method uses the predicted values of y_2t in the second stage regression to account for the endogeneity of y_2t in equation (1). By using an exclusion restriction, the 2SLS method can provide consistent estimates of the parameters of an identified equation, even if the error terms are correlated across equations. However, if the equations in the system are not identified, the 2SLS method cannot be used to estimate the parameters of the equations.
7.(c) (1) For estimating the parameters of a general structural model, describe the methods of indirect least squares (ILS) and two-stage least squares (2-SLS).
The general structural model consists of a system of simultaneous equations where some of the variables are endogenous and may be correlated with the error terms. To estimate the parameters of this model, two commonly used methods are indirect least squares (ILS) and two-stage least squares (2-SLS).
Indirect Least Squares (ILS):
The ILS method involves two steps:
In the first step, we estimate the reduced form equations for the endogenous variables. The reduced form equations express the endogenous variables as a function of the exogenous variables and the error terms from all equations in the system.
In the second step, we substitute the estimated reduced form equations into the original structural equations and estimate the parameters using ordinary least squares (OLS).
The ILS method is a consistent estimator if the structural model is exactly identified, meaning that the rank and order conditions are satisfied. However, if the structural model is over-identified or under-identified, the ILS method is not consistent.
Two-Stage Least Squares (2-SLS):
The 2-SLS method is a consistent estimator for both exactly identified and over-identified structural models. The method involves two stages:
In the first stage, we use the exogenous variables and any available instrumental variables to estimate the predicted values of the endogenous variables.
In the second stage, we substitute the predicted values of the endogenous variables into the original structural equations and estimate the parameters using OLS.
The 2-SLS method uses instrumental variables to address the endogeneity problem caused by the correlation between the endogenous variables and the error terms. Instrumental variables are exogenous variables that are correlated with the endogenous variables but uncorrelated with the error terms. By using instrumental variables, the 2-SLS method can provide consistent estimates of the parameters even if the structural model is over-identified or under-identified.
In summary, ILS and 2-SLS are two popular methods for estimating the parameters of a general structural model. The choice of method depends on the identifiability of the structural model and the availability of instrumental variables. If the model is exactly identified and instrumental variables are not available, ILS can be used. If the model is over-identified or under-identified, or instrumental variables are available, 2-SLS is a more appropriate method.
(ii) Show that for a just identified equation, the two estimators are identical.
In a just identified equation, the number of endogenous variables is equal to the number of equations in the system, so there is exactly one solution for the parameters of the equation.
Let's consider the two estimators for the parameters of a just identified equation. The first estimator is the ordinary least squares (OLS) estimator, which estimates the parameters by minimizing the sum of squared residuals from the equation:
β̂_OLS = argmin(Σ(y_t - β_0 - β_1x_t)^2)
The second estimator is the two-stage least squares (2SLS) estimator, which estimates the parameters by first regressing the endogenous variable on the exogenous variables and any available instrumental variables to obtain predicted values, and then substituting the predicted values into the original equation and estimating the parameters using OLS:
β̂_2SLS = argmin(Σ(y_t - β_0 - β_1x_t - β_2z_t)^2)
where z_t are the instrumental variables.
To show that the two estimators are identical, we need to show that β̂_OLS = β̂_2SLS.
For a just identified equation, we have exactly one solution for the parameters, so the predicted values from the first stage of 2SLS will be identical to the observed values of the endogenous variable. Therefore, we can substitute y_t for its predicted value in the second stage equation:
β̂_2SLS = argmin(Σ(y_t - β_0 - β_1x_t - β_2y_t)^2)
Taking the first derivative of the above equation with respect to β_2 and setting it equal to zero, we get:
-2Σ(y_t - β_0 - β_1x_t - β_2y_t)y_t = 0
Simplifying the above expression, we get:
Σ(y_t - β_0 - β_1x_t)y_t = β̂_OLSΣy_t
Therefore, we can write:
β̂_OLS = Σ(y_t - β_0 - β_1x_t)y_t/Σy_t
Substituting β̂_OLS into the 2SLS estimator, we get:
β̂_2SLS = argmin(Σ(y_t - β_0 - β_1x_t - β̂_OLSy_t)^2)
Taking the first derivative of the above equation with respect to β̂_OLS and setting it equal to zero, we get:
-2Σ(y_t - β_0 - β_1x_t - β̂_OLSy_t)y_t = 0
Simplifying the above expression, we get:
Σ(y_t - β_0 - β_1x_t)y_t = β̂_OLSΣy_t
which is identical to the expression we derived earlier for β̂_OLS.
Therefore, we have shown that for a just-identified equation, the two estimators, OLS and 2SLS, are identical.
If you are interested, then enroll now
Yes, I will enroll now
Thinking about it
google seo…
03topgame 03topgame;
gamesimes gamesimes;
Fortune Tiger…
Fortune Tiger…
Fortune Tiger…
EPS Machine…
EPS Machine…
seo seo;
betwin betwin;
777 777;
slots slots;
Fortune Tiger…
seo优化 SEO优化;
bet bet;
google seo…
03topgame 03topgame;
gamesimes gamesimes;
Fortune Tiger…
Fortune Tiger…
Fortune Tiger…
EPS Machine…
EPS Machine…
seo seo;
betwin betwin;
777 777;
slots slots;
Fortune Tiger…
seo优化 SEO优化;
bet bet;
google seo…
03topgame 03topgame
gamesimes gamesimes;
Fortune Tiger…
Fortune Tiger…
Fortune Tiger…
EPS машины…
Fortune Tiger…
EPS Machine…
EPS Machine…
EPP Machine…
EPS Machine…
EPTU Machine…
EPS Machine…
seo seo
谷歌seo推广 游戏出海seo,引流,快排,蜘蛛池租售;
Fortune Tiger Fortune Tiger;
Fortune Tiger Fortune Tiger;
Fortune Tiger Fortune Tiger;
Fortune Tiger Fortune Tiger;
Fortune Tiger Slots Fortune Tiger Slots;
Fortune Tiger Fortune Tiger;
Fortune Tiger Fortune Tiger;
Fortune Tiger Fortune Tiger;
Fortune Tiger Fortune Tiger;
Fortune Tiger Fortune Tiger;
Fortune Tiger Fortune Tiger;
Fortune Tiger Fortune Tiger;
Fortune Tiger Fortune Tiger;
Fortune Tiger Slots Fortune…
Fortune Tiger Slots Fortune…
Fortune Tiger Slots Fortune…
google seo google seo技术+飞机TG+cheng716051;